New machine learning tool enhances understanding of cancer mutations
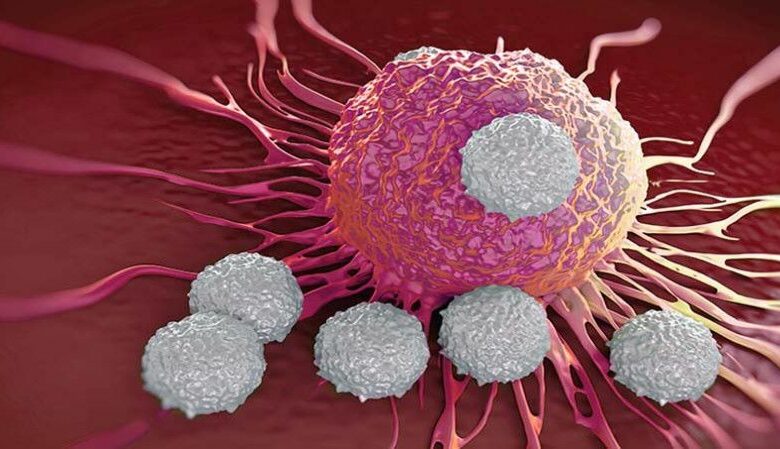
Researchers at Baylor College of Medicine have developed FunMap, a machine learning-based tool to analyze cancer-associated mutations and proteins.
In a study published in Nature Cancer, the researchers highlight FunMap’s ability to map functional relationships among 10,525 genes, leveraging data from 11 cancer types provided by the Clinical Proteomic Tumor Analysis Consortium.
FunMap identified 196,800 protein associations, offering unprecedented insights into cancer biology. Unlike previous methods, it integrates protein and mRNA data and uses supervised machine learning to enhance accuracy, outperforming traditional protein interaction networks.
According to the study, the tool uncovered new cancer drivers, such as the tumor suppressor role of genes like MAB21L4 and LGI3. For instance, MAB21L4, often underexpressed in tumors, was linked to disrupted epithelial cell differentiation—a critical factor in tumor progression.
This study demonstrates the potential of combining proteogenomics and machine learning to advance cancer research, paving the way for better therapeutic strategies and more targeted cancer treatments.