Study Reveals Historical Patterns of Atmospheric Blocking Events Using Deep Learning
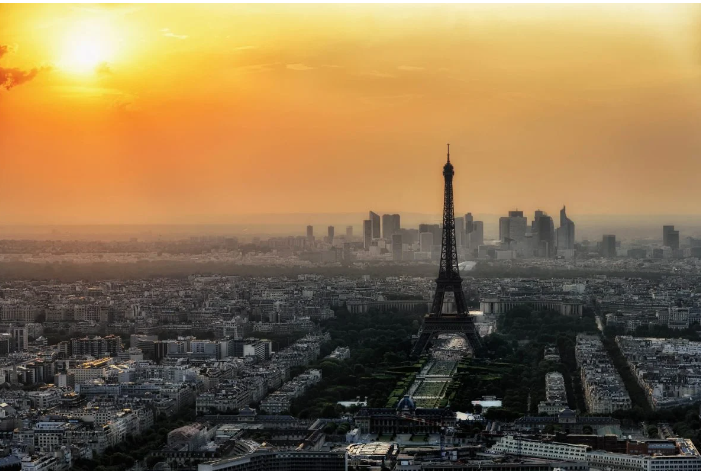
A groundbreaking study led by University of Hawai‘i at Mānoa atmospheric scientist Christina Karamperidou utilized a deep learning model to reconstruct the frequency of atmospheric blocking events over the past 1,000 years, SciTech Daily revealed yesterday.
These blocking events, characterized by stationary high-pressure systems, disrupt the jet stream and can lead to severe weather extremes such as floods and heat waves.
Karamperidou’s research highlights the relationship between blocking events and surface temperature, marking the first attempt to analyze this complex interaction over such an extended period. The deep learning model was trained using historical climate data and simulations, allowing it to infer blocking frequencies from seasonal temperature anomalies.
Understanding how climate change may influence the frequency of these events is crucial, particularly for regions like Hawai‘i, which are vulnerable to flooding linked to persistent blocking patterns. The study also emphasizes the importance of open research practices, with a web interface developed to share findings and methodologies, promoting transparency in climate science.