AI tool may detect heart, fracture risks in routine bone scans
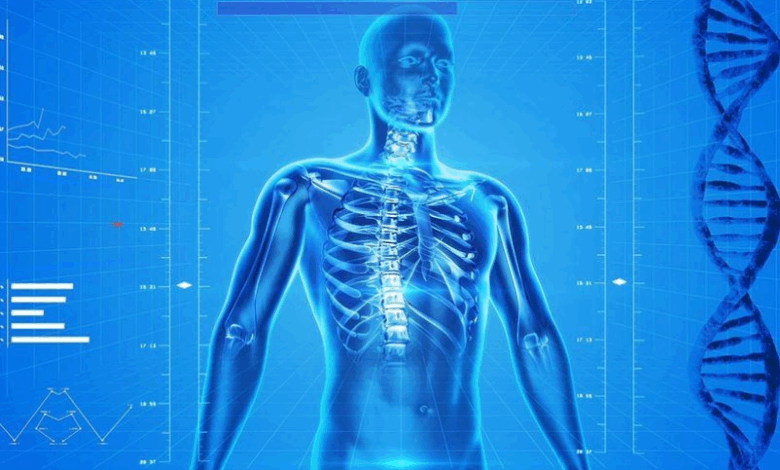
Researchers from Australia’s Edith Cowan University (ECU) and the University of Manitoba have developed a machine learning algorithm that detects cardiovascular risks and fall or fracture potential using routine bone density scans, Anadolu Agency reported.
The AI tool analyzes vertebral fracture assessment (VFA) images to identify abdominal aortic calcification (AAC), a key indicator of heart attacks, strokes, and falls.
Unlike traditional AAC assessments that take 5 to 6 minutes per image and require expert review, the new algorithm processes thousands of images in under a minute, enabling large-scale, efficient screening. ECU research fellow Dr. Cassandra Smith found that 58% of older adults tested had moderate to high AAC levels, with 25% unaware of their high cardiovascular risk.
The tool is particularly valuable for women, who are often under-screened for cardiovascular disease. ECU senior research fellow Dr. Marc Sim noted that higher AAC scores correlate with increased hospitalization from falls and fractures, highlighting the importance of vascular health in fall risk assessments. This AI innovation could transform clinical practice by providing earlier, comprehensive diagnoses during routine osteoporosis screenings.