MIT Researchers Develop Efficient Reinforcement Learning Algorithm
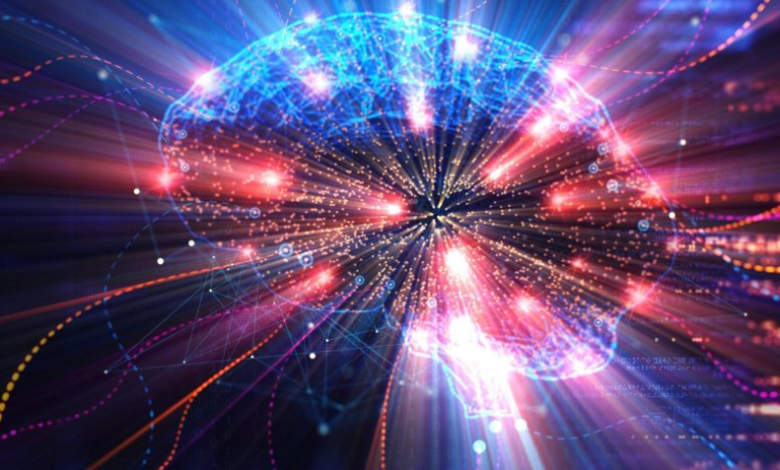
MIT researchers have unveiled a groundbreaking reinforcement learning algorithm aimed at enhancing AI decision-making in complex environments, particularly city traffic control, SciTech Daily reported yesterday.
This new method, which strategically selects optimal tasks for training, significantly boosts performance while drastically reducing data requirements—offering up to a 50-fold increase in efficiency.
The algorithm addresses common challenges in reinforcement learning, where models often struggle with slight variations in tasks, such as fluctuating traffic patterns at intersections. By focusing on a select few intersections that yield the most significant performance improvements, the researchers can maximize the algorithm’s effectiveness while minimizing training costs.
Cathy Wu, a senior author of the study, highlighted the simplicity of the algorithm, which could facilitate broader adoption within the AI community. The Model-Based Transfer Learning (MBTL) approach allows the algorithm to train independently on selected tasks, enhancing its ability to generalize across various scenarios.
Testing demonstrated that the MBTL algorithm outperformed standard methods by five to 50 times on simulated tasks, allowing it to achieve robust performance with far less data. Future research aims to extend these methods to tackle more complex real-world problems, including next-generation mobility systems.